A wealth of information can be found in server logs. They can provide you with a wealth of information on user behavior, system performance, and any problems. However, manually identifying those problems is challenging. Examining logs is necessary, but it can be challenging when there are many logs. Anomaly detection can help with it. One machine learning method that can automatically identify anomalies is anomaly detection. Within the.NET environment, developers can create robust anomaly detection systems using.NET 9 and Microsoft's open-source machine learning framework, ML.NET. Using a real-world example, we will examine the capabilities of ML.NET and how to apply it to identify issues in server logs with.NET 9.
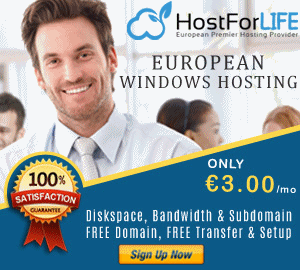
What is ML.NET?
ML.NET is a powerful, cross-platform machine learning framework designed for .NET developers. It allows you to create, train, and deploy custom machine learning models directly in C# or F# without requiring extensive data science expertise. Launched by Microsoft, ML.NET supports a wide range of scenarios.
- Classification: Binary (e.g., spam detection) or multi-class (e.g., categorizing support tickets).
- Regression: Predicting future values (e.g., sales forecasting).
- Clustering: Grouping similar data points (e.g., segmenting customers).
- Anomaly Detection: Finding outliers in datasets (e.g., identifying irregularities in server logs).
- Time-Series Analysis: Finding trends and outliers in sequential data.
- Recommendation Systems: Suggesting products or content based on user behavior.
ML.NET’s strengths include its integration with .NET tools like Visual Studio, support for pre-trained models (e.g., ONNX, TensorFlow), and the Model Builder GUI for simplified development. Whether you’re processing small datasets or scaling to enterprise-level applications, ML.NET offers flexibility and performance, making it an ideal choice for embedding intelligence into .NET 9 projects.
Project Overview: Detecting Error Spikes in Server Logs
We’re going to create a .NET 9 console application that uses ML.NET to find unusual activity in server log data. We’re especially looking at error counts over time. This example is like a real-world situation where a sudden increase in errors could mean there’s a problem with the server. This would let administrators fix the problem before it gets worse.
Step 1. Setting Up the Environment
Please find the complete source code: Click Here
To begin, ensure you have,
- .NET 9 SDK: Installed from the official Microsoft site.
- Visual Studio Code (or Visual Studio): For coding and debugging. I will be using VS Code for this project.
- ML.NET Packages: Added via NuGet.
Let’s start. If you have C# Dev Kit, then you can create the project in VS Code or use below command.
Create a new console application.
dotnet new console -n AnomalyDetection -f net9.0
cd AnomalyDetection
Add the ML.NET NuGet packages.
dotnet add package Microsoft.ML
dotnet add package Microsoft.ML.TimeSeries

Step 2. Defining the Data Models.
Create a Models folder and add two classes.
LogData.cs: Represents server log entries with timestamps and error counts.
namespace AnomalyDetection.Models;
public record LogData
{
public DateTime Timestamp { get; set; }
public float ErrorCount { get; set; }
}
AnomalyPrediction.cs: Represents the model’s output, indicating whether an anomaly is detected.
using Microsoft.ML.Data;
namespace AnomalyDetection.Models;
public record AnomalyPrediction
{
[VectorType(3)]
public double[] Prediction { get; set; } = [];
}
AnomalyResult.cs: Represents the output result from the model.
namespace AnomalyDetection.Models;
public record AnomalyResult
{
public DateTime Timestamp { get; set; }
public float ErrorCount { get; set; }
public bool IsAnomaly { get; set; }
public double ConfidenceScore { get; set; }
}
Step 3. Implementing Anomaly Detection Logic with ML.NET
Create a Services folder and add AnomalyDetector.cs.
using System;
using AnomalyDetection.Models;
using Microsoft.ML;
namespace AnomalyDetection.Services;
public class AnomalyDetectionTrainer
{
private readonly MLContext _mlContext;
private ITransformer _model;
public AnomalyDetectionTrainer()
{
_mlContext = new MLContext(seed: 0);
TrainModel();
}
private void TrainModel()
{
// Simulated training data (in practice, load from a file or database)
var data = GetTrainingData();
var dataView = _mlContext.Data.LoadFromEnumerable(data);
// Define anomaly detection pipeline
var pipeline = _mlContext.Transforms.DetectIidSpike(
outputColumnName: "Prediction",
inputColumnName: nameof(LogData.ErrorCount),
confidence: 95.0, // Double instead of int
pvalueHistoryLength: 5
);
// Train the model
_model = pipeline.Fit(dataView);
}
public List<AnomalyResult> DetectAnomalies(List<LogData> logs)
{
var dataView = _mlContext.Data.LoadFromEnumerable(logs);
var transformedData = _model.Transform(dataView);
var predictions = _mlContext.Data.CreateEnumerable<AnomalyPrediction>(transformedData, reuseRowObject: false);
return logs.Zip(predictions, (log, pred) => new AnomalyResult
{
Timestamp = log.Timestamp,
ErrorCount = log.ErrorCount,
IsAnomaly = pred.Prediction[0] == 1,
ConfidenceScore = pred.Prediction[1]
}).ToList();
}
//dummy training data
private List<LogData> GetTrainingData()
{
return new List<LogData>(){
new() { Timestamp = DateTime.Now.AddHours(-5), ErrorCount = 2 },
new() { Timestamp = DateTime.Now.AddHours(-4), ErrorCount = 3 },
new() { Timestamp = DateTime.Now.AddHours(-3), ErrorCount = 2 },
new() { Timestamp = DateTime.Now.AddHours(-2), ErrorCount = 50 }, // Anomaly: Spike!
new() { Timestamp = DateTime.Now.AddHours(-1), ErrorCount = 4 },
new() { Timestamp = DateTime.Now.AddHours(-6), ErrorCount = 2 }
};
}
}
Explanation
_mlContext: An instance of MLContext, the core ML.NET object for managing data, models, and transformations. It’s initialized with a seed (0) for reproducible results.
_model: An ITransformer object that holds the trained anomaly detection model, applied later for predictions.
private void TrainModel()
{
var data = GetTrainingData();
var dataView = _mlContext.Data.LoadFromEnumerable(data);
var pipeline = _mlContext.Transforms.DetectIidSpike(
outputColumnName: "Prediction",
inputColumnName: nameof(LogData.ErrorCount),
confidence: 95.0,
pvalueHistoryLength: 5
);
_model = pipeline.Fit(dataView);
}
Purpose: Trains the anomaly detection model using simulated data.
Steps
- Data Loading: Calls GetTrainingData to fetch dummy server log data, then converts it into an IDataView using LoadFromEnumerable.
- Pipeline Definition: Uses DetectIidSpike from MLContext.Transforms to create a pipeline for detecting anomalies in independent and identically distributed (IID) data:
- outputColumnName: “Prediction”: Names the output column for anomaly results.
- inputColumnName: nameof(LogData.ErrorCount): Specifies the input data (error counts).
- confidence: 95.0: Sets a 95% confidence level for anomaly detection.
- pvalueHistoryLength: 5: Defines a sliding window of 5 data points to evaluate anomalies.
- Training: Fits the pipeline to the data, producing a trained _model.
DetectAnomalies Method
public List<AnomalyResult> DetectAnomalies(List<LogData> logs)
{
var dataView = _mlContext.Data.LoadFromEnumerable(logs);
var transformedData = _model.Transform(dataView);
var predictions = _mlContext.Data.CreateEnumerable<AnomalyPrediction>(transformedData, reuseRowObject: false);
return logs.Zip(predictions, (log, pred) => new AnomalyResult
{
Timestamp = log.Timestamp,
ErrorCount = log.ErrorCount,
IsAnomaly = pred.Prediction[0] == 1,
ConfidenceScore = pred.Prediction[1]
}).ToList();
}
Step 4. Check the anomalies in the logs with the above service
Update Program.cs
using AnomalyDetection.Models;
using AnomalyDetection.Services;
//create a dummy log data
var logs = new List<LogData>(){
new() { Timestamp = DateTime.Now.AddHours(-5), ErrorCount = 2 },
new() { Timestamp = DateTime.Now.AddHours(-4), ErrorCount = 3 },
new() { Timestamp = DateTime.Now.AddHours(-3), ErrorCount = 2 },
new() { Timestamp = DateTime.Now.AddHours(-2), ErrorCount = 50 },
new() { Timestamp = DateTime.Now.AddHours(-1), ErrorCount = 4 },
new() { Timestamp = DateTime.Now.AddHours(-6), ErrorCount = 2 }
};
//create an instance of the AnomalyDetectionTrainer
var trainer = new AnomalyDetectionTrainer();
//detect anomalies
var results = trainer.DetectAnomalies(logs);
//print the results
foreach (var result in results)
{
Console.WriteLine($"Timestamp: {result.Timestamp}, ErrorCount: {result.ErrorCount}, IsAnomaly: {result.IsAnomaly}, ConfidenceScore: {result.ConfidenceScore}");
}
Let's run the console app and validate the results.
The project structure is as shown below.

A wealth of information can be found in server logs. They can provide you with a wealth of information on user behavior, system performance, and any problems. However, manually identifying those problems is challenging. Examining logs is necessary, but it can be challenging when there are many logs. Anomaly detection can help with it. One machine learning method that can automatically identify anomalies is anomaly detection. Within the.NET environment, developers can create robust anomaly detection systems using.NET 9 and Microsoft's open-source machine learning framework, ML.NET. Using a real-world example, we will examine the capabilities of ML.NET and how to apply it to identify issues in server logs with.NET 9.